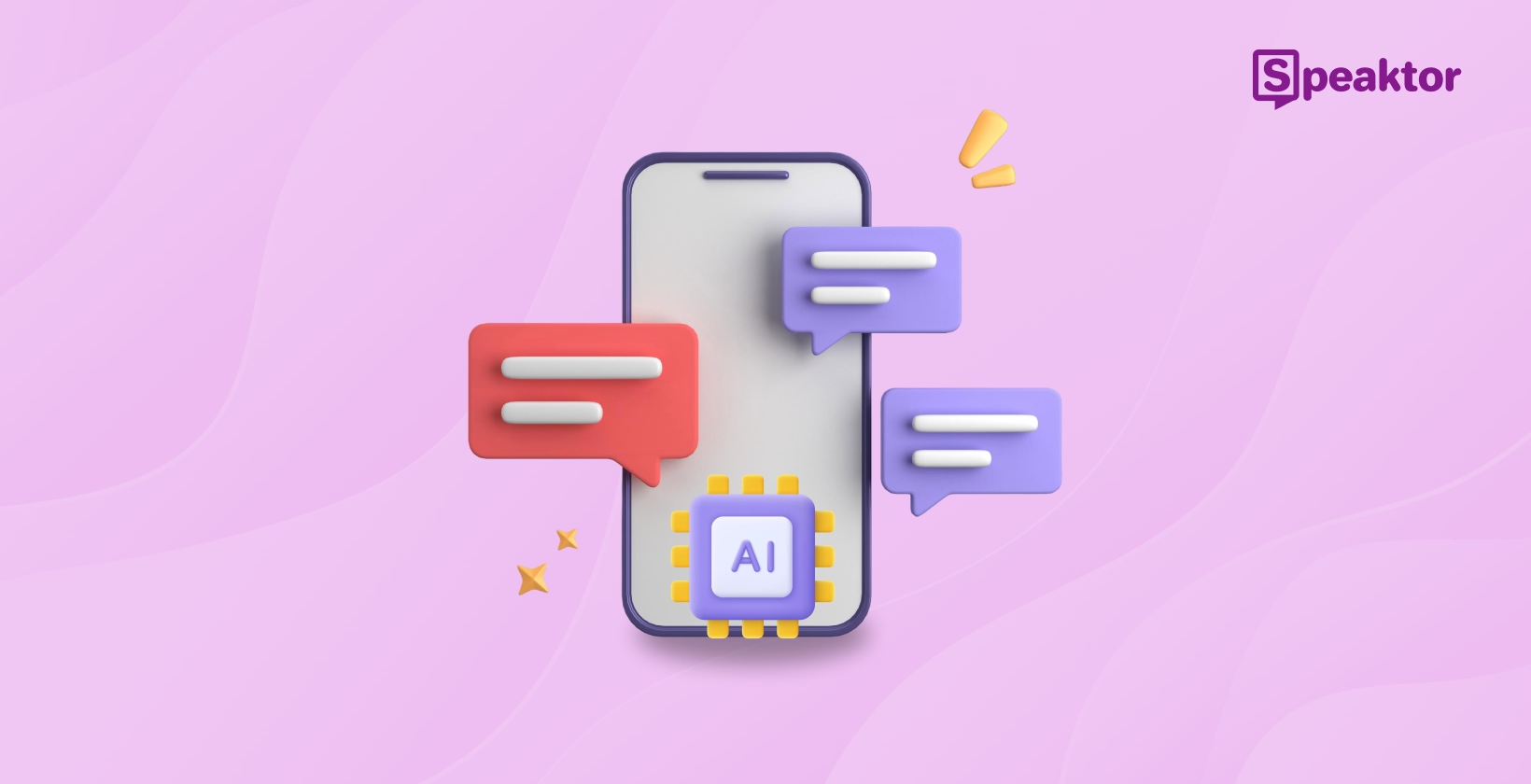
Conversational AI: Definition, Importance, and Techniques
Turn Texts into Speech and Read Aloud
Conversational AI technology has revolutionized customer support systems, replacing traditional channels like phone calls and emails with intelligent, responsive virtual assistants. Businesses increasingly implement conversational AI solutions to deliver personalized services across all customer touchpoints, available 24/7 without interruption. According to Gartner research, conversational AI will handle over 70% of customer interactions by 2027, demonstrating the rapid adoption of this transformative technology in customer service applications.
In this blog, we'll explore the fundamental components of conversational AI systems, examine how these intelligent platforms process information through natural language processing, and investigate real-world applications transforming industries today.
What Is Conversational AI?
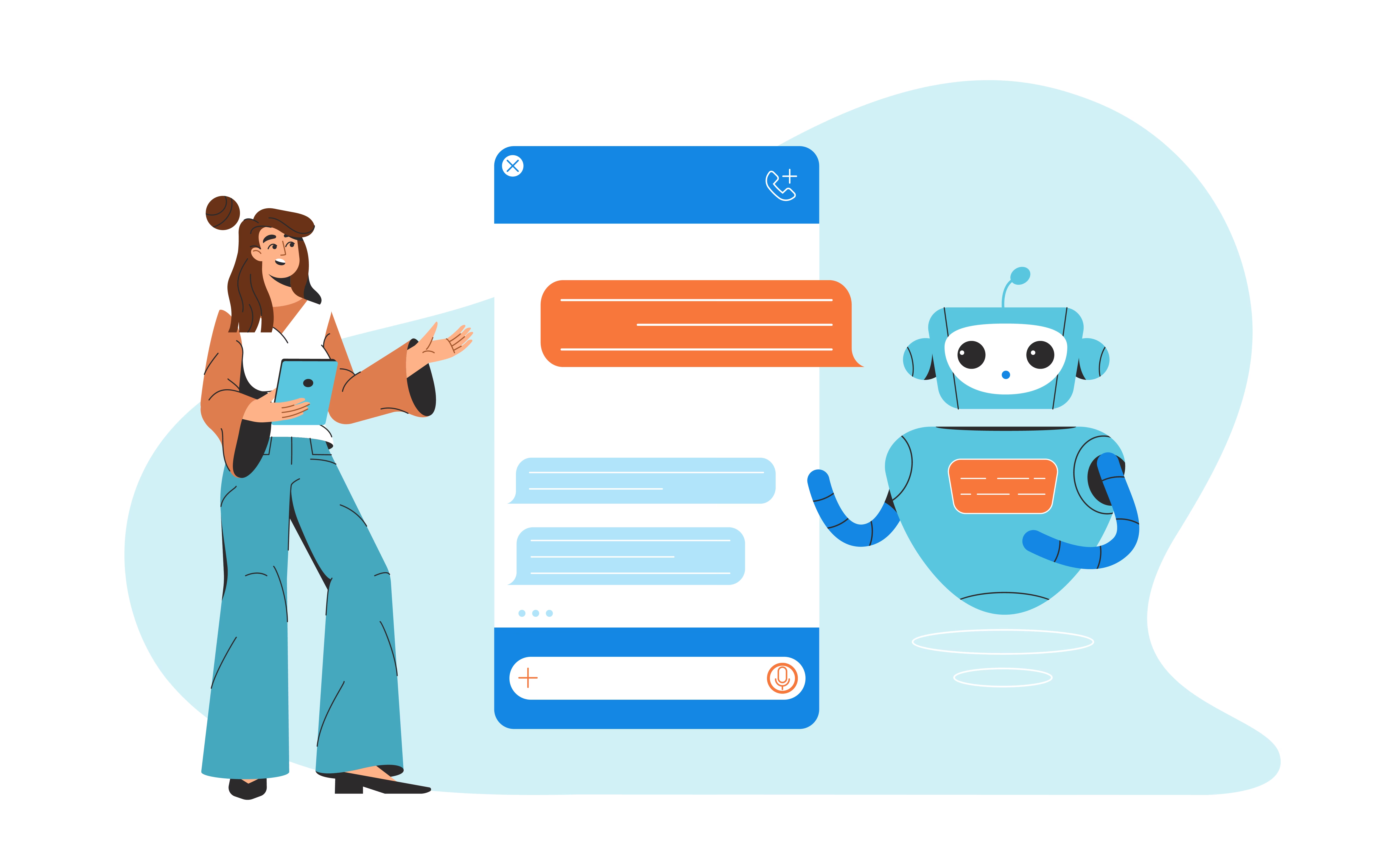
Conversational AI represents advanced artificial intelligence systems that engage in natural, human-like conversations with users. These systems process text or speech inputs, understand user intent through context analysis, and generate relevant responses in real-time while continuously learning from each interaction.
The evolution of conversational AI has progressed from simple rule-based chatbots like ELIZA in the 1960s to today's sophisticated systems. Modern conversational AI, much like in AI dubbing, utilizes natural language processing, deep learning, and cloud computing to provide contextual understanding and personalized responses. AI virtual assistants like Siri, Alexa, and Google Assistant have expanded this technology beyond text with the integration of advanced AI voices, making conversational AI an integral part of daily life.
Core Components of Conversational AI
Behind effective AI chatbots lies a framework of technologies working together to understand and respond to human conversations. These components form the foundation of modern conversational AI systems:
Natural Language Processing (NLP)
NLP enables conversational AI to interpret human language in its natural form. When users send messages or speak commands, NLP breaks down this language to determine meaning and intent. This technology helps AI recognize user needs even with unusual phrasing, using techniques like tokenization, intent recognition, and sentiment analysis. Advanced NLP models track conversation history to maintain context across exchanges, enabling more natural interactions.
Machine Learning in AI Systems
Machine learning gives conversational AI systems the ability to improve over time. Rather than using rigid scripts, these systems train on datasets of real conversations, learning how people naturally communicate. Through ongoing interactions, conversational AI refines its understanding, adapting to new language variations, slang, and regional dialects to create increasingly responsive experiences.
Voice Recognition Technology
Voice recognition technology (ASR) is essential for voice-based conversational assistants. It converts spoken language into text that AI can process through NLP. Modern ASR systems achieve high accuracy using deep learning trained on diverse speech samples, adapting to different accents, speaking speeds, and background noise for reliable voice interactions across varied environments.
How Does Conversational AI Work?
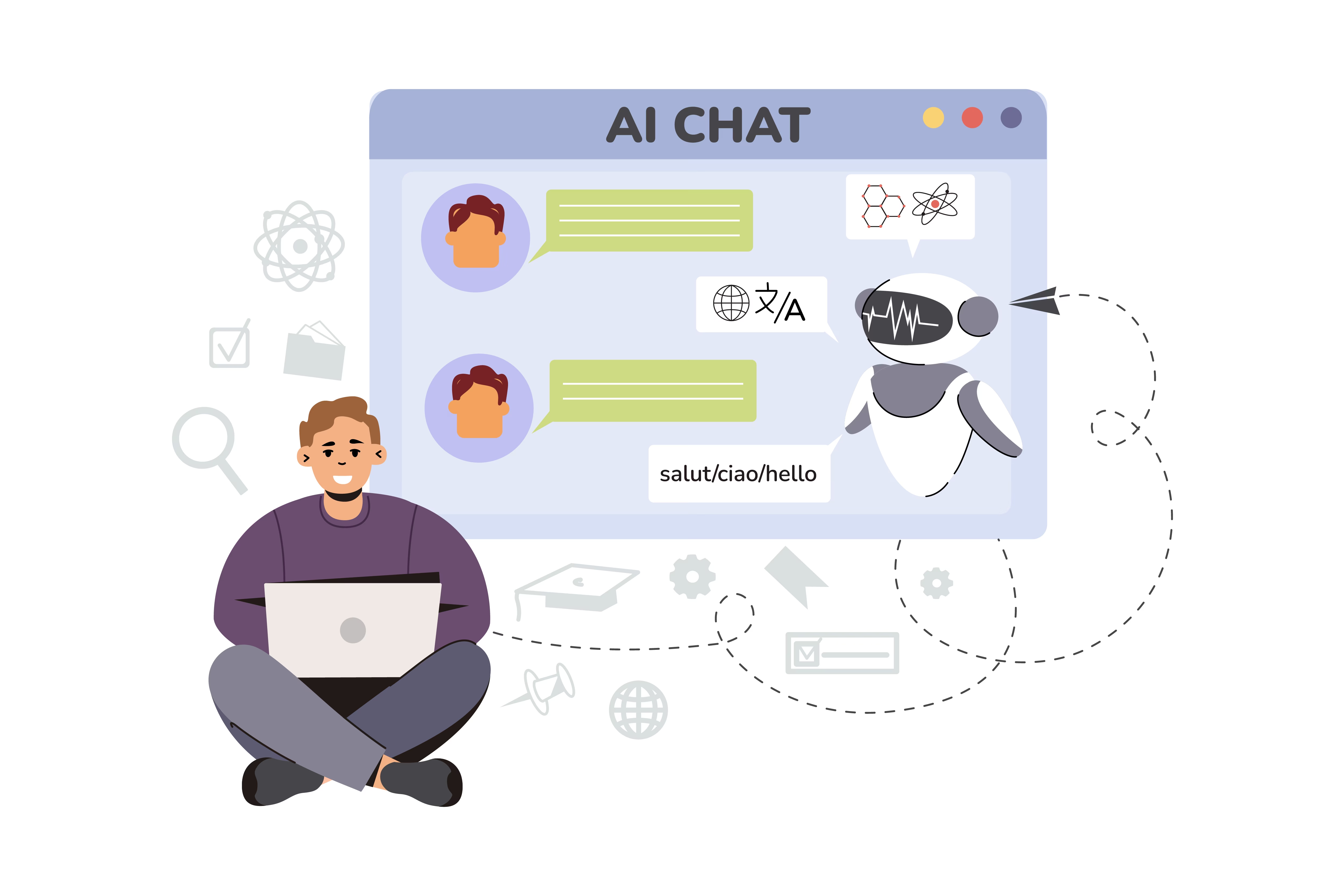
Conversational AI systems follow a structured workflow to understand, interpret, and respond to user requests. This process operates through three primary phases—input processing, response generation, and output delivery—each powered by specialized language models, machine learning algorithms, and speech processing technologies.
The Input Phase
The input phase initiates when users engage with conversational AI through text messages or voice commands directed at intelligent voice assistants. For text-based systems, AI directly analyzes written input, while voice-based interactions require preliminary speech-to-text conversion through ASR technology.
Once input becomes available in processable format, the NLP system performs comprehensive analysis to identify key information elements:
- Critical keywords indicating subject matter
- Underlying user intent driving the request
- Emotional sentiment conveyed through language choices
- Contextual relationship to previous conversation elements
Advanced conversational AI maintains contextual awareness throughout interactions. These systems retain relevant details from earlier exchanges, enabling them to answer follow-up questions and manage multi-turn dialogues with natural conversation flow mirroring human interaction patterns.
The Processing Phase
After understanding user requests, conversational AI enters the processing phase where response determination occurs. AI language models, particularly large language models (LLMs), generate responses by predicting the most contextually appropriate and natural replies based on identified user intent and accumulated conversation history.
Many conversational systems incorporate predefined decision trees and conversation flows for structured interactions such as appointment scheduling or order processing. These frameworks ensure consistent handling of common scenarios while maintaining natural language interaction quality.
The Output Phase
In the final phase, conversational AI delivers responses to users through either text display or synthesized speech. Text responses appear directly within chat interfaces, while voice interactions utilize text-to-speech technology to convert generated text into natural-sounding speech output.
Modern text-to-speech engines create increasingly human-like vocal responses with appropriate intonation, rhythm, and emotional qualities. This advanced output technology contributes significantly to creating seamless conversation experiences that approximate natural human communication patterns.
Real-World Applications of Conversational AI
Conversational AI has transformed human-computer interaction across both consumer and business environments. From virtual assistants to customer service chatbots, these applications have become increasingly common in daily life.
AI Virtual Assistants in Daily Life
AI virtual assistants like Amazon Alexa, Google Assistant, and Apple's Siri have become essential tools for millions of users. Through simple voice commands, these systems manage daily tasks from setting reminders to controlling smart home devices.
Smart home integration represents a major growth area for conversational AI. According to Statista, smart home technology will reach 92.5% of households by 2029, with AI assistants becoming central hubs for managing connected devices through intuitive voice interfaces.
Business Applications of Conversational AI
In business environments, AI chatbots now handle millions of customer service interactions daily. These automated systems provide instant support without human intervention, improving efficiency while maintaining service quality.
Bank of America's AI assistant Erica demonstrates this impact effectively, processing over 1.5 billion client interactions since launch. E-commerce platforms like Amazon and Sephora use conversational AI to deliver personalized shopping recommendations based on customer history, enhancing user experience and increasing conversion rates.
Top Text-to-Speech Tools for Conversational AI
Modern conversational AI delivers responses to users through either text display or synthesized speech. Text-based responses are shown directly in chat interfaces, while voice interactions utilize text to speech technology for converting text into natural-sounding speech outputs. These tools transform written content into natural-sounding speech, enhancing accessibility and engagement across various applications.
Top text-to-speech solutions include:
- Speaktor - Versatile multilingual platform with extensive voice customization
- Google Text-to-Speech - Widely integrated solution with broad language support
- Amazon Polly - Cloud-based service with neural voice technology
- IBM Watson Text to Speech - Enterprise solution with emotion detection
- Microsoft Azure Text to Speech - Comprehensive platform with translation capabilities
Comparison of Top Text-to-Speech Platforms
Tool | Key Strength | Best For | Languages | Voice Options | Affordable |
---|---|---|---|---|---|
Speaktor | Versatility | Content creation | 50+ | 100+ | ✅ Yes |
Google TTS | Integration | Accessibility | 40+ | 220+ | ✅ Yes |
Amazon Polly | Neural voices | Audiobooks | 60+ | 50+ | ❌ No |
IBM Watson | Security | Enterprise use | 30+ | Limited | ❌ No |
Microsoft Azure | Translation | Call centers | 45+ | Limited | ❌ No |
Speaktor
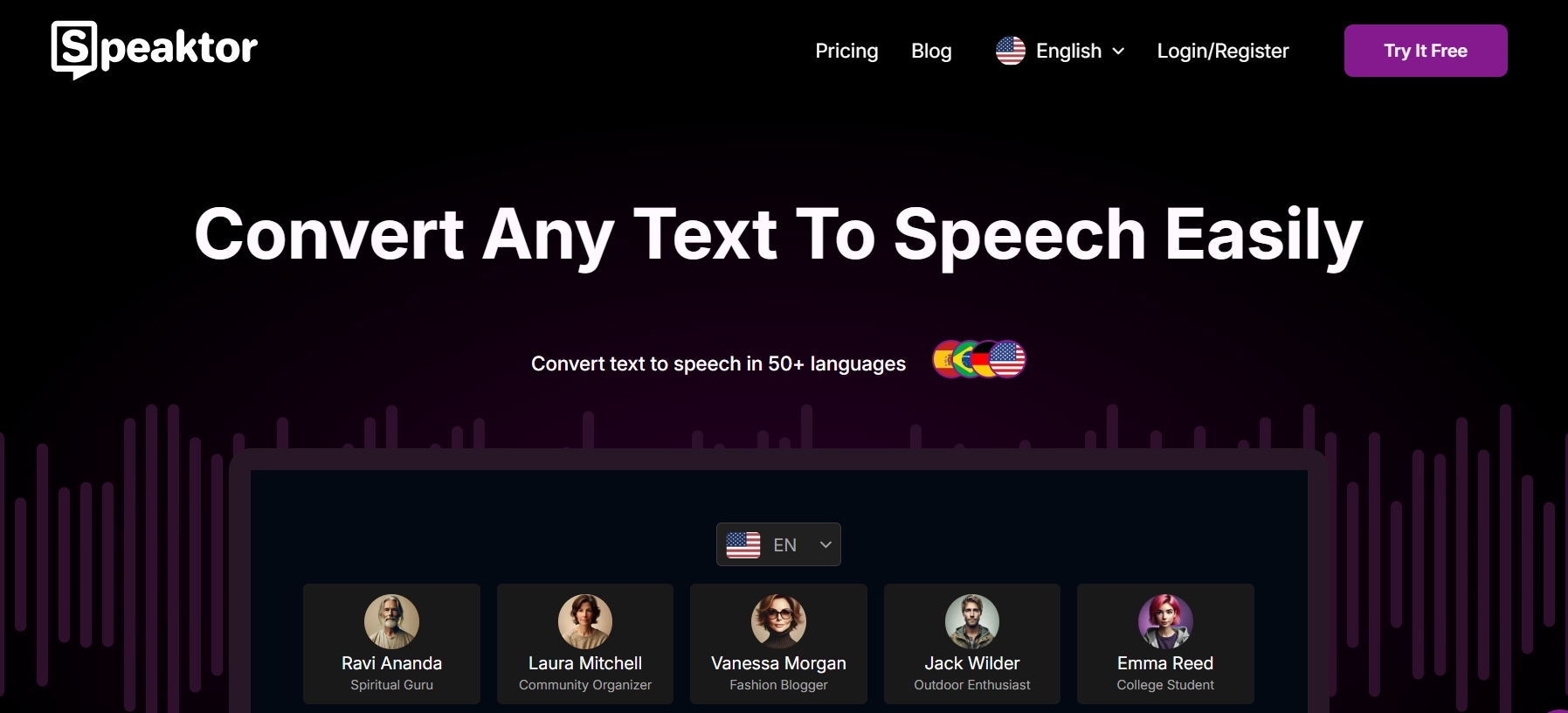
Speaktor delivers advanced text-to-speech technology with remarkably human-like output for content creators, businesses, educators, and accessibility advocates.
Pros:
- Supports over 50 languages for global content creation
- Offers 100+ voice options with different styles and tones
- Multiple download formats (MP3, WAV, MP3+TXT, WAV+TXT)
- Processes text from various sources (direct input, documents, PDFs, images)
- Platform-agnostic with cloud storage integration
Cons:
- Newer to market than some competitors
- May require internet connection for full functionality
- Advanced features may require paid subscription
Speaktor enhances accessibility for individuals with visual impairments while improving productivity through automated voiceover creation that saves significant time and resources.
How Speaktor Works
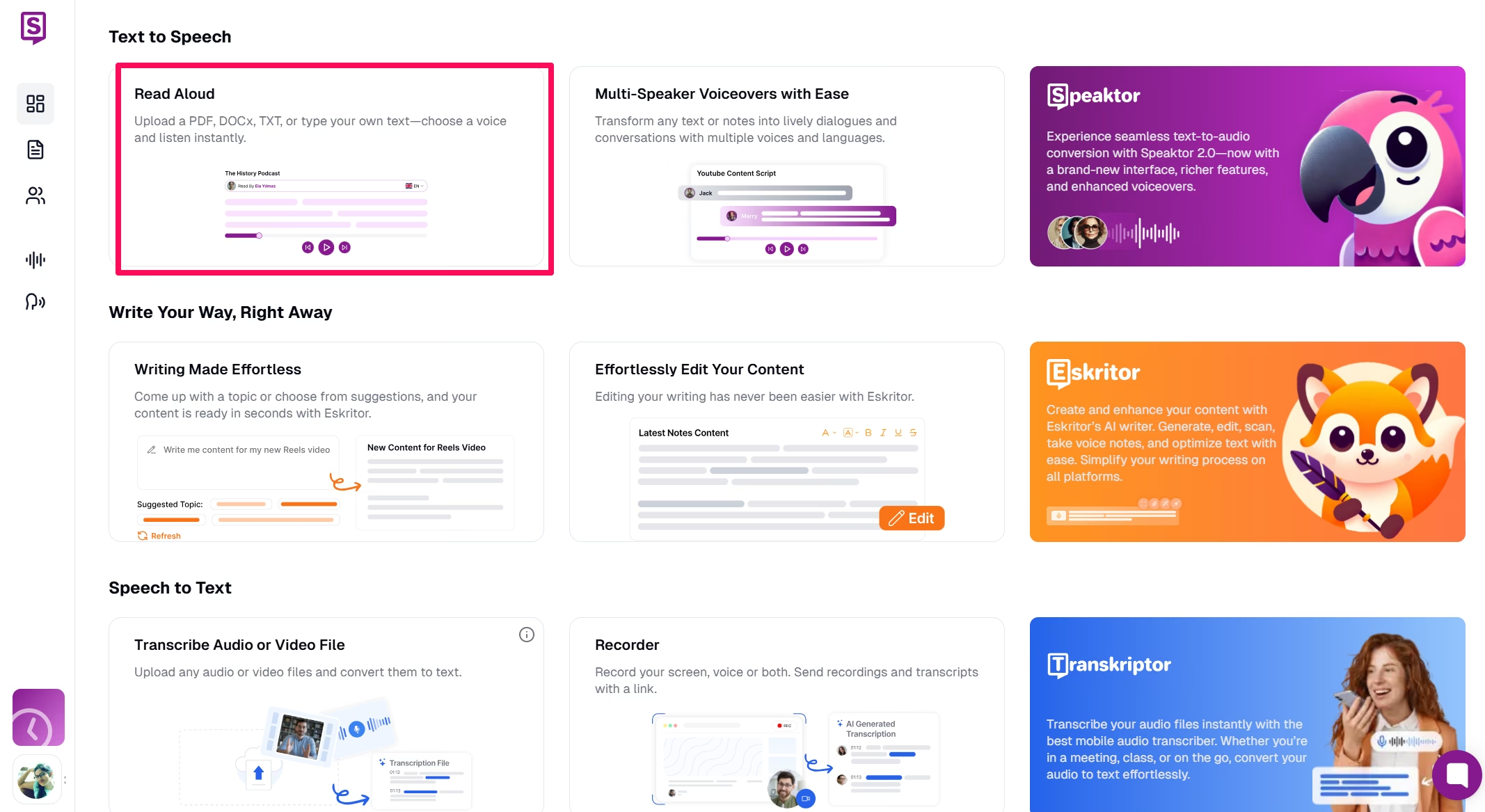
Speaktor uses a streamlined workflow:
- Upload or input text content
- Select language from supported options <image5>
- Choose voice characteristics
- AI processes text to generate natural speech
- Download or integrate the completed audio <image6>
Google Text-to-Speech
Google's Text-to-Speech is integrated throughout Android devices, Google Assistant, and accessibility features with over 220 voices across 40+ languages.
Pros:
- Extensive language and voice support
- WaveNet voices for natural speech patterns
- Seamless integration with Google ecosystem
- Free for basic usage and accessibility purposes
Cons:
- Advanced features require Cloud TTS API (paid)
- Limited customization compared to enterprise solutions
- Less control over voice characteristics
Google TTS excels in accessibility applications while providing developers with implementation tools through the Cloud Text-to-Speech API.
Amazon Polly
Amazon Polly provides cloud-based text-to-speech using deep learning for natural-sounding output, ideal for audiobooks, virtual assistants, and customer support.
Pros:
- Neural voice technology for lifelike speech
- SSML support for precise control over speech characteristics
- Real-time streaming capabilities
- Seamless AWS integration
Cons:
- Higher pricing compared to alternatives
- Requires AWS knowledge for optimal implementation
- Best features limited to paid tiers
The platform excels in SSML support, allowing precise control over pronunciation, volume, pitch, and speaking rate while delivering enterprise-grade reliability.
IBM Watson Text to Speech
IBM Watson's Text to Speech offers enterprise-focused solutions with custom voice training, emotion-based speech modulation, and secure deployment options.
Pros:
- Superior pronunciation accuracy for specialized terminology
- Emotion detection capabilities
- Enterprise-grade security features
- Advanced customization options
Cons:
- Higher cost structure
- More complex implementation
- Fewer voice options than some competitors
Watson TTS particularly excels in industries with specific vocabulary requirements such as healthcare, finance, and technology while creating nuanced interactions that respond appropriately to user emotional states.
Microsoft Azure Text to Speech
Microsoft Azure Text to Speech delivers custom neural voice development, multilingual support, and real-time translation within Microsoft's AI ecosystem.
Pros:
- Custom Neural Voice feature for brand-specific voices
- Excellent translation capabilities
- Integration with other Azure services
- Strong enterprise support
Cons:
- Higher price point
- Requires Azure ecosystem knowledge
- Complex for small implementations
Azure TTS is particularly valuable for call centers, e-learning platforms, and assistive technologies while enabling comprehensive AI solution development combining multiple conversational technologies.
Future Trends in Conversational AI
Conversational AI continues evolving rapidly with several key developments on the horizon:
- Multimodal AI will process text, voice, images, and video simultaneously, allowing AI assistants to interpret facial expressions and emotional cues for more natural interactions.
- Autonomous AI agents will shift from reactive to proactive capabilities, independently executing complex tasks without constant human guidance. OpenAI's Auto-GPT exemplifies this trend toward self-directing AI systems.
- Within five years, conversational AI will approach indistinguishability from human interactions in many contexts, with AI assistants evolving into autonomous, emotionally intelligent digital agents capable of handling approximately 95% of customer support interactions.
Conclusion
Conversational AI fundamentally transforms human-computer interaction by creating more natural, efficient communication channels. As AI capabilities advance, increasingly sophisticated systems will seamlessly integrate into daily routines, providing intuitive interfaces for digital interaction. Organizations implementing these solutions gain significant advantages through improved customer experiences and operational efficiency.
While numerous text-to-speech platforms exist today, Speaktor distinguishes itself through exceptional ease of use, natural voice quality, and comprehensive multilingual support. Whether for content creation, accessibility enhancement, or business automation, Speaktor delivers seamless AI-powered audio solutions for diverse implementation needs. Experience the transformative capabilities of advanced conversational AI speech technology—explore Speaktor today!
Frequently Asked Questions
Conversational AI refers to artificial intelligence systems that enable human-like interactions through text or voice. These systems use technologies like natural language processing (NLP), machine learning (ML), and speech recognition to understand and respond to user queries in real time.
Regular chatbots only follow pre-set rules and can’t answer anything outside of those rules. Conversational AI, however, can understand meaning, ask follow-up questions, and improve with experience. This makes it more helpful and realistic in conversations.
Conversational AI works in three steps. First, it listens to or reads what a person says. Then, it figures out the meaning using a smart brain called machine learning. Finally, it responds with text or speech, just like a real conversation. It gets better over time by learning from past interactions.
Most conversational AI tools follow strict privacy rules to protect user data. However, some AI assistants collect information to improve their services, so it’s important to check privacy settings. Many companies use encryption and security measures to keep AI conversations safe.